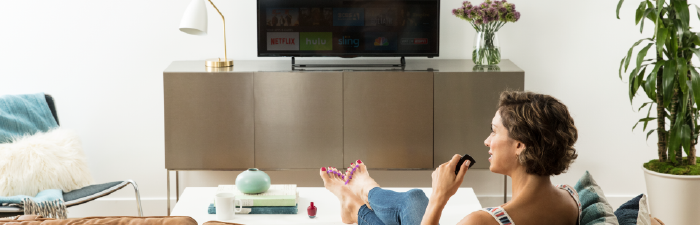
Artificial Intelligence (AI) is quickly becoming the most anticipated component of devices coming to market today. Between Bose’s new voice-supported smart speakers, Google launching voice-controlled shopping in the UK, and Amazon letting developers create their own Alexa-powered gadgets, it’s a safe bet that the best of AI and voice control products is yet to come.
But as we discussed in a blog post last month, this technology is not without its flaws – and the industry is not without big challenges. While consumer adoption rates of AI products are on the uptick – 1 in 6 U.S. adults currently own a voice-enabled device – that doesn’t necessarily mean they’ll be forgiving if those products don’t work well. AI needs a generous amount of machine learning and training in actual home environments with real people to provide a seamless user experience.
Here’s where Customer Validation (CV) comes in. By getting a functional product into the hands of real customers in their real environments, you can gain critical insight into user behaviors and bypass some of these obstacles before launch. In particular, Field Testing (the third phase of CV) enables you to get a closer look at product adoption before it goes into the wild.
Why Field Testing for AI?
Field Testing focuses on collecting tester responses to your product in an unguided, natural use context over an extended period of time. The more observational structure of this test type lends itself to examining behavioral, attitudinal, and longitudinal trends related to feature adoption.
During a Field Test, users submit ongoing feedback following either a cadenced or event-based schedule. A cadenced schedule is ideal for returning feedback about the sum of your testers’ product experiences over a certain amount of time. If, for example, you’ve developed a smart thermostat, you may ask field testers to submit product feedback once a week for six weeks.
By contrast, an event-based schedule lends itself to differentiating between experiences with feedback. Examples of this could include using the A/C for the first time, how the product responds when the temperature drops below 60°F, etc. In either case, testers record their experiences through journals. Allowing your testers’ impressions and observations to surface naturally is the closest thing you’ll get to the at-home experience of your customers.
Questions Addressed by Field Testing:
+ What features do my customers use frequently?
+ Which features do my customers ignore?
+ How long do my customers stay interested in my product? Days? Weeks?
+ How is my product learning from my customers?
+ How are my customers adapting and responding to my product over time?
Collecting user data over a stretch of time also allows you to analyze and hone your machine learning algorithms. By testing your product, early adopters are also training it. Their feedback gives context to your analytics, painting an entire picture of user interaction. Through product analytics, usage, and user feedback, Field Testing returns both quantitative and qualitative data.
The more exposure your product has to testers in these natural use cases, the more evolved it will be by the time you launch. This head start will better enable you to provide personalized experiences for your customers, which is enormously valuable: 59% of consumers in their 20s and 30s are likely to spend more for a service specifically customized to their usage patterns. In addition, the flow of tester feedback from Field Tests helps build out a framework for future releases.
Why Not Beta Testing?
If Field Tests are so useful, then why doesn’t everyone run them? As the last phase of Customer Validation (which is often accounted for late in the game, after the product schedule is already set), Field Testing tends to fall off due to a lack of time or resources. To supplement this, we’ve seen many teams try to fold Field Test objectives into their Beta Test efforts – usually with limited success.
Unlike Field Tests, Beta Tests guide users through a feature-focused tour to assess product satisfaction. Since they are, by design, very directed – Beta Tests work well because they focus on specific features, after all – they don’t offer an accurate portrayal of natural use. Testers might, for example, spend time on features they wouldn’t typically use, in ways they wouldn’t typically use them. What’s more, the two to three week time frame of the average Beta Test doesn’t really lend itself to long-term machine learning. Without adequate time and natural use scenarios, tacking Field Test objectives onto your Beta Test is really just teaching your product to adapt to Beta Testers, not to your larger audience.
What You Can Do
If you’re planning to test your product but you don’t have the resources for a Field Test this time around, there are still ways you can beneficially experiment with it. For instance, you can plan for a week of unstructured testing during your next Beta Test. While it might not be as effective as a traditional Field Test, diversifying tester activities is interesting for your testers. It also allows certain issues to come up organically. It’s a useful way to dip your toe into the kind of insights you see from Field Tests without compromising your Beta Test results.
You can learn more about Field Testing, including strategies for planning tests and recruiting ideal testers, in our on-demand webinar, How to Evaluate Feature Adoption Through Field Testing.