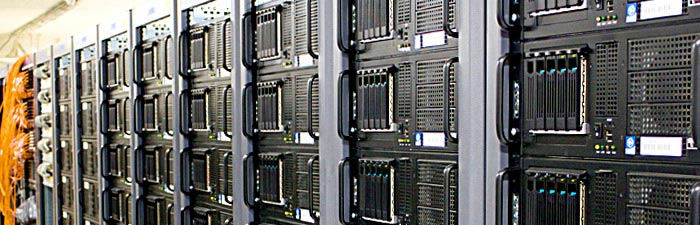
Generally, we try to keep our blog posts focused on the here and now. Our primary goal is to give people practical advice on how to manage better beta tests. Sometimes, though, it’s interesting to think about possible futures for beta testing. And recent research from HP on data mining unstructured textual data, like social media and product reviews, is great material for that kind of thinking.
First off, a little clarification is in order. Big Beta isn’t a term we’ve used before, but there’s more to it than just a catchy blog post title. Large consumer and enterprise companies tend to approach beta testing a little differently. They’re more likely to invest in beta as a large-scale program with an entire staff dedicated to its operations. They also tend to have more formal integrations, like single sign-on systems linking their beta management platform (preferably Centercode) to existing customer communities. Thus, to the extent that there’s a “Big Data” future for beta testing, it will most likely start with Big Beta.
What is HP doing that sounded so interesting to us? It’s more complex than they can reasonably describe in a blog post and slide deck, but here’s the gist. HP has invested in researching ways to add computationally-useful meaning and structure to things like social media messages and product reviews. This allows them to feed the underlying meaning of messages into data mining/machine learning algorithms, which are then analyzed as part of a bigger picture.
To borrow from their example, consider a Twitter post like, “I really like this HP 6510 printer, but the paper jams are really frustrating.” HP’s research helps them to automatically infer that this message is positive about the Photosmart 6510 overall but negative about the paper tray. If these inferences are put into some sort of data structure (e.g., assigning +1 and -1 scores in a matrix of pre-determined parameters), they become much easier for a computer to work with. Analysts then have the capability to cross-reference these types of data points against other streams, like sales information and support tickets, to look for correlations and interesting insights. This ultimately provides them with real-time actionable data that they can use to make faster decisions and smarter responses.
So, how does all this relate back to beta testing? Well, for starters, beta testing is a great source for both unstructured and structured data. One of the fascinating things about HP’s research is that they’re now able to merge these unstructured data streams with structured historical data, like customer demographics, data fields from support tickets, etc. to create a much deeper understanding. In beta, unstructured text (feedback reports, daily journals, forum posts) and structured data (bug severity scales, survey responses, user profiles) are constantly being generated together in potentially useful ways.
One possibility would be to use data from beta testing to train supervised learning algorithms that can later be relied upon by the real-time systems. In a beta test, feedback classification is both very important and very easy. It’s trivial to have a beta tester select what aspect of the product a bug report relates to (e.g., the paper tray). Alternatively, beta test managers can work to classify feedback on the back end as part of their feedback management tasks. Either way, classification provides certainty. You can use properly classified data to identify phrases and descriptions customers are more likely to use when describing a paper tray problem, making future predictions more accurate.
Another idea would be to use what’s learned from the real-time systems in the other direction, benefiting the beta program. Insights generated for released products can help beta managers better understand the significance and best course of action for problems and praise encountered during their tests. If certain words, tone, sentiment, etc. correlated strongly with major problems in a released product right before a drop in sales or string of bad reviews, then you now have something you can watch out for algorithmically during beta tests to set off the alarm bells and dive deeper into.
What HP’s research shows us is that these types of ideas aren’t pie-in-the-sky anymore. A future where the walls between beta testing and business intelligence are non-existent is very exciting for us, and we’re looking forward to seeing the ways Big Beta uses Centercode to interact with Big Data. To learn more about the tools and processes driving Customer Validation, check out the Customer Validation Industry Report.
Get the Customer Validation Industry Report
Image courtesy of Flickr user torkildr.