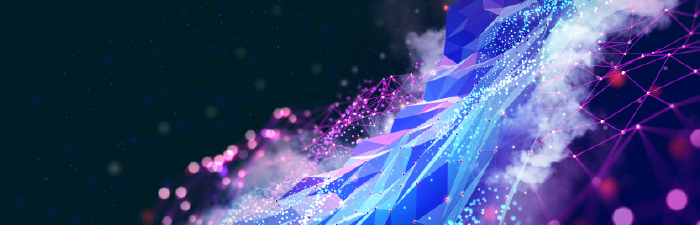
Why does AI that performs so well in a lab underperform when it’s out in the real world? Earlier this month, a team of 40 Google researchers published a study about just that — and their investigation returned some vital insights into the challenges of developing machine learning.
Research points to a phenomenon known as underspecification, where seemingly similar models in a lab ripple out to massive variations in practice. The differences between the models are so small and random that they’re easily overlooked. In the end, these tiny differences make it near-impossible to tell which models will actually work in the real world.
Combined with data shift — the mismatch between the data used to train the AI and the data encountered in real-world use — there’s a lot of room for error. And if you’re a maker of tech that relies on machine learning, these errors aren’t doing you or your customers any favors.
So what can you do about this? The leader of the research team said it best: “We need to be doing a lot more testing.”
Using Testers to Combat Big Challenges
Using beta testers to validate machine learning algorithms before release is essential to understanding how your tech performs in the real world. But that only solves part of the problem: it tells you when there’s something wrong but not necessarily how to solve it.
To start fixing the other half, you need to fix the way your AI is trained. That involves getting your hands on a lot more real-world data before release to both create a machine learning model and test it on an ongoing basis.
Here’s where your beta testers can step up to the plate again. Target market testers are a wellspring of nuanced, in-the-wild use cases. In addition to the detailed, qualitative feedback testers give during beta and delta, the constant flow of sensor and usage data provides a big, market-aligned data set for training machine learning before release.
Who’s Doing It Right
One prime example of this large scale data collection effort is Tesla. The automaker uses data voluntarily collected from customers and employees (a fleet about half-a-million strong) to feed its Autopilot and Full Self-Driving systems. As of late October 2020, Elon Musk reported that Tesla had released Full Self-Driving beta software to a select group of its fleet.
This move is consistent with their focus on “a fundamental architectural rewrite of our neural networks and control algorithms.”
In August 2020, iRobot, the company behind the well-known mobile vacuum cleaner the Roomba, announced an AI-powered platform known as iRobot Genius Home-Intelligence.
Similar to Tesla, iRobot collected tens of thousands of images from inside homes (with users’ permission, of course) to develop their machine learning vision. In fact, iRobot’s CEO Colin Angle boasted that the company’s fleet of data collecting vehicles is “probably second only to Tesla’s.”
While we can’t all be Elon Musk (and that’s probably for the best), these are just two examples in a sea of areas where the abundance of data made available by targeted beta testers give deep learning and neural networks a boost. With a growing number of industries tapping into machine learning and AI to improve both functionality and user experience, how proactive a company is in training (or retraining) their machine learning algorithms will define its future success.
As AI-powered abilities — computer vision; pattern, image, and speech recognition; natural language processing (NLP); and prediction, to name a few — play a greater and greater role in the tech of today and tomorrow, your customers will be invaluable in fueling real-world AI performance.
How Centercode Helps
Improving your machine learning products starts with growing your community of targeted testers. We got you. Here at Centercode, we’ve helped some of the most iconic tech companies (like iRobot) increase their beta tester communities to refine their products. Read about these industry-specific use cases (and other ways Centercode connects you to your customers) in our ebook, Making Good Technology Great.